是否可以使用matplotlib scikit-learn分类报告进行绘图?我们假设我打印分类报告如下:
print '\n*Classification Report:\n', classification_report(y_test, predictions)
confusion_matrix_graph = confusion_matrix(y_test, predictions)
我得到:
Clasification Report:
precision recall f1-score support
1 0.62 1.00 0.76 66
2 0.93 0.93 0.93 40
3 0.59 0.97 0.73 67
4 0.47 0.92 0.62 272
5 1.00 0.16 0.28 413
avg / total 0.77 0.57 0.49 858
我如何"绘制"avobe图表?
1> Franck Derno..:
扩展Bin的答案:
import matplotlib.pyplot as plt
import numpy as np
def show_values(pc, fmt="%.2f", **kw):
'''
Heatmap with text in each cell with matplotlib's pyplot
Source: /sf/ask/17360801/
By HYRY
'''
from itertools import izip
pc.update_scalarmappable()
ax = pc.get_axes()
#ax = pc.axes# FOR LATEST MATPLOTLIB
#Use zip BELOW IN PYTHON 3
for p, color, value in izip(pc.get_paths(), pc.get_facecolors(), pc.get_array()):
x, y = p.vertices[:-2, :].mean(0)
if np.all(color[:3] > 0.5):
color = (0.0, 0.0, 0.0)
else:
color = (1.0, 1.0, 1.0)
ax.text(x, y, fmt % value, ha="center", va="center", color=color, **kw)
def cm2inch(*tupl):
'''
Specify figure size in centimeter in matplotlib
Source: /sf/ask/17360801/
By gns-ank
'''
inch = 2.54
if type(tupl[0]) == tuple:
return tuple(i/inch for i in tupl[0])
else:
return tuple(i/inch for i in tupl)
def heatmap(AUC, title, xlabel, ylabel, xticklabels, yticklabels, figure_RdBu'):
'''
Inspired by:
- /sf/ask/17360801/
- /sf/ask/17360801/
'''
# Plot it out
fig, ax = plt.subplots()
#c = ax.pcolor(AUC, edgecolors='k', linedashed', linewidths=0.2, cmap='RdBu', vmin=0.0, vmax=1.0)
c = ax.pcolor(AUC, edgecolors='k', linedashed', linewidths=0.2, cmap=cmap)
# put the major ticks at the middle of each cell
ax.set_yticks(np.arange(AUC.shape[0]) + 0.5, minor=False)
ax.set_xticks(np.arange(AUC.shape[1]) + 0.5, minor=False)
# set tick labels
#ax.set_xticklabels(np.arange(1,AUC.shape[1]+1), minor=False)
ax.set_xticklabels(xticklabels, minor=False)
ax.set_yticklabels(yticklabels, minor=False)
# set title and x/y labels
plt.title(title)
plt.xlabel(xlabel)
plt.ylabel(ylabel)
# Remove last blank column
plt.xlim( (0, AUC.shape[1]) )
# Turn off all the ticks
ax = plt.gca()
for t in ax.xaxis.get_major_ticks():
t.tick1On = False
t.tick2On = False
for t in ax.yaxis.get_major_ticks():
t.tick1On = False
t.tick2On = False
# Add color bar
plt.colorbar(c)
# Add text in each cell
show_values(c)
# Proper orientation (origin at the top left instead of bottom left)
if correct_orientation:
ax.invert_yaxis()
ax.xaxis.tick_top()
# resize
fig = plt.gcf()
#fig.set_size_inches(cm2inch(40, 20))
#fig.set_size_inches(cm2inch(40*4, 20*4))
fig.set_size_inches(cm2inch(figure_width, figure_height))
def plot_classification_report(classification_report, title='Classification report ', cmap='RdBu'):
'''
Plot scikit-learn classification report.
Extension based on /sf/ask/17360801/
'''
lines = classification_report.split('\n')
classes = []
plotMat = []
support = []
class_names = []
for line in lines[2 : (len(lines) - 2)]:
t = line.strip().split()
if len(t) <2: continue
classes.append(t[0])
v = [float(x) for x in t[1: len(t) - 1]]
support.append(int(t[-1]))
class_names.append(t[0])
print(v)
plotMat.append(v)
print('plotMat: {0}'.format(plotMat))
print('support: {0}'.format(support))
xlabel = 'Metrics'
ylabel = 'Classes'
xticklabels = ['Precision', 'Recall', 'F1-score']
yticklabels = ['{0} ({1})'.format(class_names[idx], sup) for idx, sup in enumerate(support)]
figure_width = 25
figure_height = len(class_names) + 7
correct_orientation = False
heatmap(np.array(plotMat), title, xlabel, ylabel, xticklabels, yticklabels, figure_width, figure_height, correct_orientation, cmap=cmap)
def main():
sampleClassificatiOnReport= """ precision recall f1-score support
Acacia 0.62 1.00 0.76 66
Blossom 0.93 0.93 0.93 40
Camellia 0.59 0.97 0.73 67
Daisy 0.47 0.92 0.62 272
Echium 1.00 0.16 0.28 413
avg / total 0.77 0.57 0.49 858"""
plot_classification_report(sampleClassificationReport)
plt.savefig('test_plot_classif_report.png', dpi=200, format='png', bbox_inches='tight')
plt.close()
if __name__ == "__main__":
main()
#cProfile.run('main()') # if you want to do some profiling
输出:
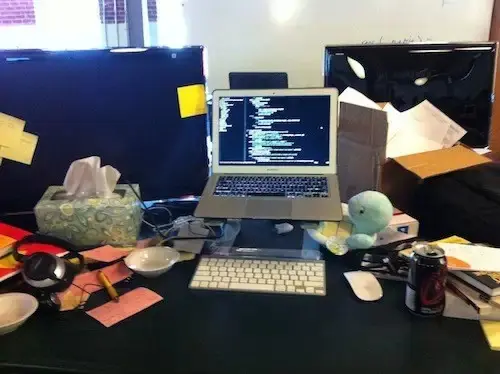
更多类(~40)的示例:

当前版本的`matplotlib`似乎无法使用所述解决方案.行`ax = pc.get_axes()`必须改为`ax = pc.axes`.
如果没有itertools,请删除“从itertools导入izip”,然后用zip替换izip。
但是为什么要使用izip?它比zip慢并且与Python3不兼容:https://stackoverflow.com/questions/32659552/izip-not-working-in-python-3-x
2> Bin..:
我刚刚为此编写了一个函数plot_classification_report()
.希望能帮助到你.该函数取出了classification_report函数的put作为参数并绘制得分.这是功能.
def plot_classification_report(cr, title='Classification report ', with_avg_total=False, cmap=plt.cm.Blues):
lines = cr.split('\n')
classes = []
plotMat = []
for line in lines[2 : (len(lines) - 3)]:
#print(line)
t = line.split()
# print(t)
classes.append(t[0])
v = [float(x) for x in t[1: len(t) - 1]]
print(v)
plotMat.append(v)
if with_avg_total:
aveTotal = lines[len(lines) - 1].split()
classes.append('avg/total')
vAveTotal = [float(x) for x in t[1:len(aveTotal) - 1]]
plotMat.append(vAveTotal)
plt.imshow(plotMat, interpolation='nearest', cmap=cmap)
plt.title(title)
plt.colorbar()
x_tick_marks = np.arange(3)
y_tick_marks = np.arange(len(classes))
plt.xticks(x_tick_marks, ['precision', 'recall', 'f1-score'], rotation=45)
plt.yticks(y_tick_marks, classes)
plt.tight_layout()
plt.ylabel('Classes')
plt.xlabel('Measures')
对于您提供的示例classification_report.这是代码和输出.
sampleClassificatiOnReport= """ precision recall f1-score support
1 0.62 1.00 0.76 66
2 0.93 0.93 0.93 40
3 0.59 0.97 0.73 67
4 0.47 0.92 0.62 272
5 1.00 0.16 0.28 413
avg / total 0.77 0.57 0.49 858"""
plot_classification_report(sampleClassificationReport)

以下是如何将其与sklearn classification_report输出一起使用:
from sklearn.metrics import classification_report
classificatiOnReport= classification_report(y_true, y_pred, target_names=target_names)
plot_classification_report(classificationReport)
使用此功能,您还可以将"平均/总计"结果添加到绘图中.要使用它,只需添加如下参数with_avg_total
:
plot_classification_report(classificationReport, with_avg_total=True)
3> larrywgray..:
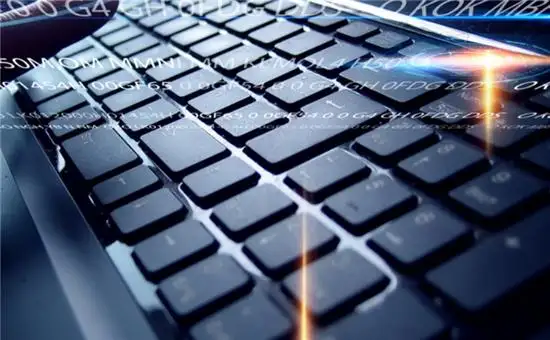
我的解决方案是使用python包,Yellowbrick.简而言之,Yellowbrick将scikit-learn与matplotlib相结合,为您的模型生成可视化效果.在几行中,您可以执行上面建议的操作.
http://www.scikit-yb.org/en/latest/api/classifier/classification_report.html
from sklearn.naive_bayes import GaussianNB
from yellowbrick.classifier import ClassificationReport
# Instantiate the classification model and visualizer
bayes = GaussianNB()
visualizer = ClassificationReport(bayes, classes=classes, support=True)
visualizer.fit(X_train, y_train) # Fit the visualizer and the model
visualizer.score(X_test, y_test) # Evaluate the model on the test data
visualizer.poof() # Draw/show/poof the data